ENSAM has recognized expertise in the construction of parametric solutions associated with parametrized ordinary and partial differential equations. As soon as such parametric solutions are available, the response of a model, for a given choice of parameters, can be evaluated in almost real-time, opening numerous opportunities for online engineering. Thus, optimization, inverse identification, uncertainty propagation, and real-time control can proceed under the stringent real-time constraint, making a possible efficient diagnosis, prognosis, and decision-making. When the predictions differ from measurements performed on the physical asset, it can be concluded that the model under consideration is unable to efficiently describe the reality. In that case, a data-driven model is elaborated by using advanced physics-aware machine learning to fill the gap between nominal model predictions and measurements, the so-called model of “ignorance”. When combining both models, the physics-based and the data-driven, the so-called hybrid-twin results. These technologies: parametric model solution, model enrichment from data and the hybridization of both, will be applied within the GENEX project to complex materials in processing conditions, and more particularly to thermoplastic and thermoset resins, involved in advanced composites processing, enabling address all the multi-physics content along all the involved scales, as for example reticulation and crystallization driven by the thermal and mechanical history.
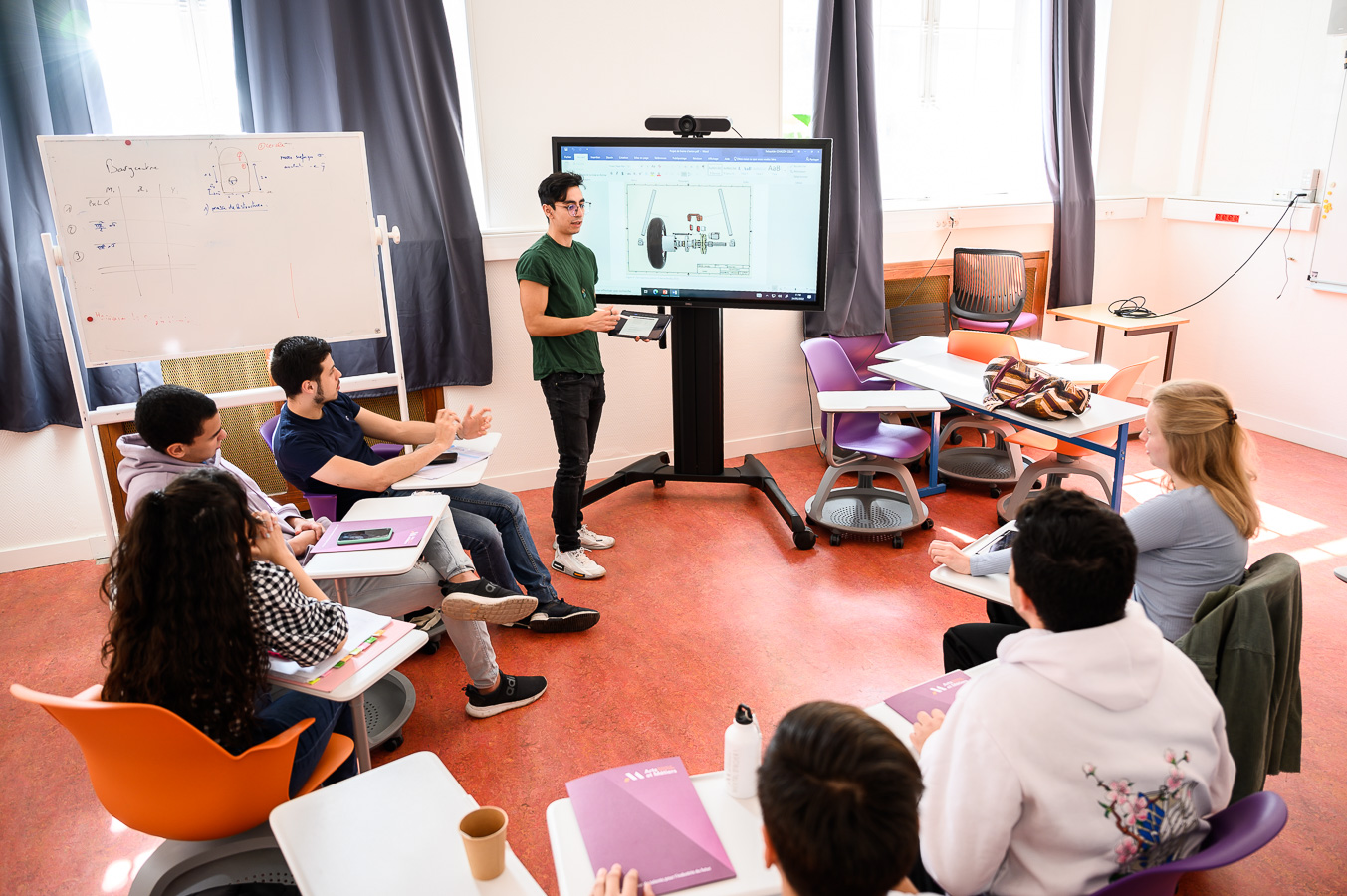